Instead of starting from “how do we hire top talent?”, start from “what are our weaknesses?”
Why are you hiring? Are you hiring to do more, or are you hiring to achieve more?
Design your hiring process to find the right people to strengthen your teams’ weaknesses, rather than trying to find the best people.
Instead of “how can we find the smartest people?” think about “how can we find people who will make our team stronger?”
Often the language used by those hiring betrays their view of people as resources. Or, to use its current popular disguise: “talent”.
“We hire top talent” “the candidate didn’t meet our bar”, “our hiring funnel selects for the best”. “we hire smart people and get out of their way”. We design “fair tests” that are an “objective assessment” of how closely people match our preconceptions of good.
The starting point for the talent mindset is that we want to hire the smartest people in the “talent pool”. If only we can hire all the smartest people, that will give us a competitive advantage!
If you focus on hiring brilliant people, and manage to find people who are slightly smarter on average than your competitors, will you win? Achievements in tech seldom stem solely from the brilliance of any one individual. Successes don’t have a single root cause any more than failures do.
We’re dealing with such complex systems; teams that can capture and combine the brilliance of several people will win.
With the right conditions a team can be smarter than any individual. With the wrong conditions a team can be disastrously wrong; suffering from over confidence and groupthink, or infighting and undermining each other. Hiring the right people to help create the right conditions in the team gives us a better chance of winning than hiring the smartest people.
Talent Mindset | Weaknesses Mindset |
Find top Talent | Find skills that unlock our potential |
Grow Team | Transform Team |
Help teams do more things | Help teams achieve greater things |
People who can do what we’re doing | People can do things we can’t do |
Hire the best person | Hire the person who’s best for the team |
People who match our biased idea of good | People who have what we’re missing |
Fair tests & Objective assessment | Equal opportunity to demonstrate what they’d add to our team |
Consistent process | Choose your own adventure |
Hire the most experienced/skilled candidate | Hire the person who’ll have the greatest impact on the team’s ability. |
Number of candidates & Conversion rate | How fast can we move for the right candidate? |
Centralised Process & Bureaucracy | Teams choose their own people |
Grading candidates | Collaborating with candidates |
Prejudging what a good candidate looks like | Reflecting on our team’s weaknesses |
Fungibility | Suitability |
Smart people | People who make the team smarter |
Intelligence | Amplifying others |
Culture Fit | Missing Perspectives |
People who’ve accomplished great things | People who’ll unblock our greatness |
Exceptional individuals. | People we can grow and who will grow us |
What didn’t the candidate demonstrate against checklist | What would the candidate add to our team |
Talent-oriented hiring
If we start out with the intent to find the “best people” it will shape our entire process.
We write down our prejudices about what good looks like in job descriptions. We design a series of obstacles^Winterviews to filter out candidates who don’t match these preconceptions. Those who successfully run the gauntlet are rewarded with an offer, then we figure out how to best put them to use.
Hiring processes are centralised to maximise efficiency & throughput. We look at KPIs around the number of candidates at each stage in the funnel, conversion rates.
Interviewing gets shared out around teams like a tax that everyone pays into. Everyone is expected to interview for the wider organisation.
Hiring committees are formed and processes are standardised. We try to ensure that every candidate is assessed as fairly as possible, against consistent criteria.
We pat ourselves on the back about how we only hire the top 1% of applicants to our funnel. We’re hiring “top talent” and working here is a privilege. We’re the best of the best.
Weaknesses-oriented hiring
There’s another approach. Instead of starting from our idea of talent and the strengths we’re looking for, start from our weaknesses. What’s missing in our team that could help us achieve more?
Maybe we have a team of all seniors, frequently stuck in analysis. We need some fresh perspectives and bias for action.
Maybe we are suffering from groupthink and need someone with a different background and new perspectives. Someone who can help generate some healthy debate?
Maybe we have all the technical skills we need but nobody is skilled at facilitating discussions. We struggle to get the best out of the team.
Perhaps we’re all fearful of a scaling challenge to come. We could use someone experienced who can lend the team some courage to meet the challenge.
Maybe those in the existing team specialise in frontend tech, and we’ve realised we need to build and run a data service. We could learn to do it, but bringing someone in with existing knowledge will help us learn faster.
Maybe we are all software engineers, but are spending most of our time diagnosing and operating production systems. Let’s add an SRE in the team.
Maybe we don’t even know what our weaknesses are—until we experience collaboration with the right person who shows us how to unlock our potential.
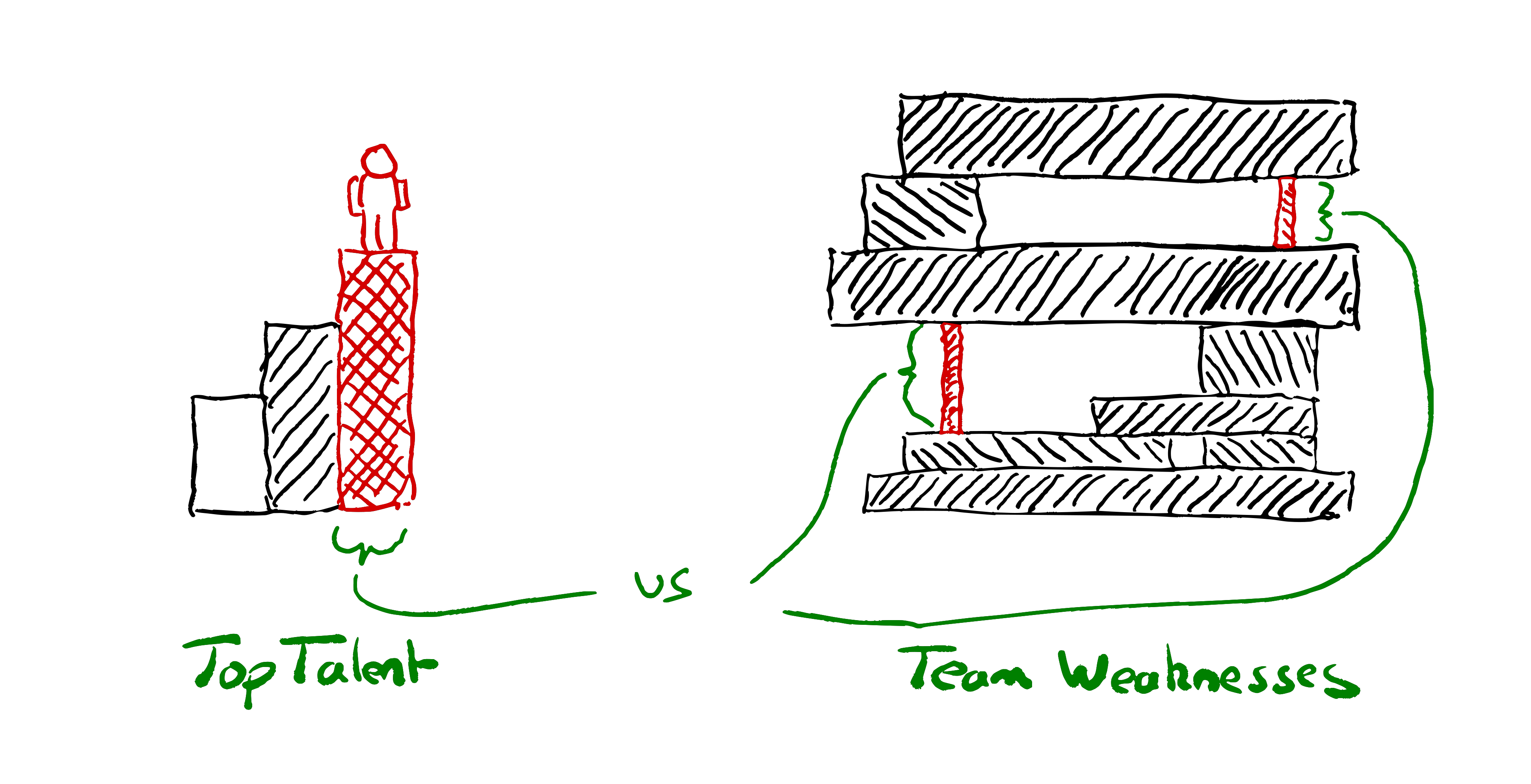
Identify your weaknesses. Use them to draft role descriptions and design your interview processes.
Design your interviews to assess what the candidate brings to your team. How do they reduce your weaknesses and what strengths would they add?
Leave space in the process to discover things people could bring to your team that you weren’t aware you needed.
A talent-oriented process would assess how the candidate stacks up against our definition of good. A weaknesses-oriented process involves collaborating with the candidate, to see whether (and how) they might make your team stronger.
If possible, have candidates join in with real work with the team. When this is not feasible for either side, design exercises that allow people on your team to collaborate with them.
Pair together on a problem. Not what many companies call paired interviews where it’s really an assessor watching you code under pressure. Instead, really work together to solve something. Help the candidate. Bounce ideas off each other. Share who’s actually doing the typing, as you would if you were pairing with a colleague. Don’t judge if they solved the same problems you solved; see what you can achieve together.
A weaknesses-oriented process might mean saying no to someone eminently qualified and highly skilled; because you have their skills well covered in the team already.
A weaknesses-oriented process might mean saying yes to someone inexperienced who’s good at asking the right questions. Someone whose feedback will help the experienced engineers in the team keep things simple and operable.
Why not both?
It’s often worth thinking about when something good is not appropriate. There are rarely “best practices”, only practices that have proven useful in a given context.
At scale I can see the need for the talent-oriented hiring approach in addition to weaknesses-oriented.
Exposing all of your teams’ variety of hiring needs to candidates may create too much confusion.
You may well want a mechanism to ensure some similarity. A mechanism to select for those who share the organisational values. To find those with enough common core skills to increase the chances that they can move from team to team. Indeed, long-lived and continuously funded teams are not a given in all organisations.
If you’re getting thousands of applications a day you’ll probably want a mechanism to improve signal to noise ratio for teams wishing to hire. Especially if you don’t want to use education results as a filter.
I suspect a lot of hiring dysfunction comes from people copying the (very visible) talent-oriented top-of-funnel hiring practices that big companies use. Copy-pasting as the entire hiring mechanism for their smallish team.
Start from reflecting on your weaknesses. Whose help could you use? Some of the practices from the talent-oriented approach may be useful, but don’t make them your starting point. Start from your weaknesses if you want strong teams.